
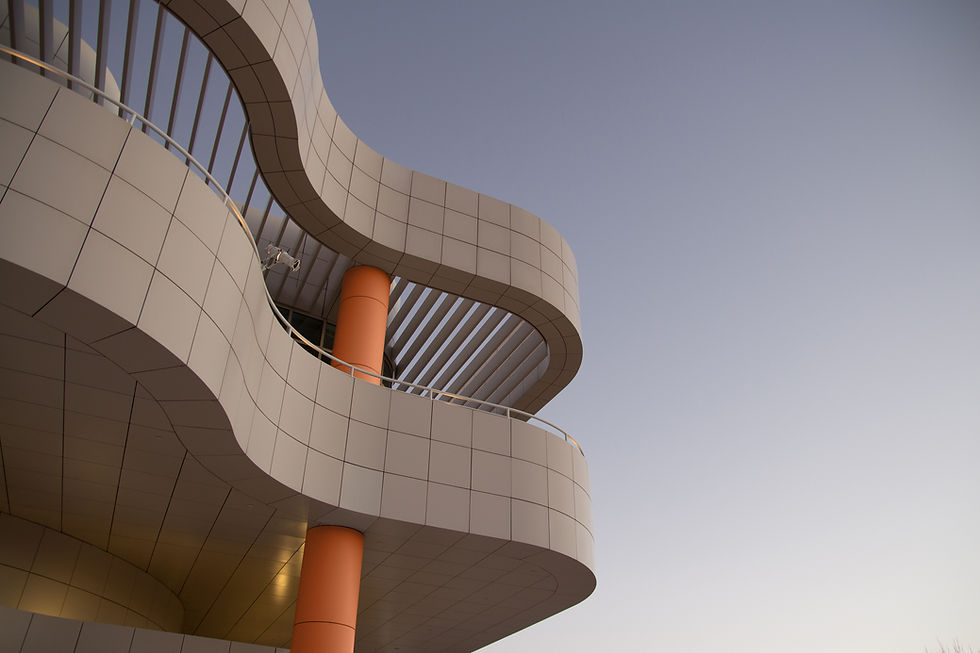
AI, or artificial intelligence, is a fairly nebulous term that is rarely given a satisfying definition. You might hear definitions saying something like, “artificial intelligence is any computer system that attempts to replicate human-level reasoning.” That’s a good definition for what AI is designed to accomplish, but it doesn’t actually tell us anything about how AI is different from traditional computing.
Ultimately, there’s another, also deceptively simple explanation for what makes AI, AI. Any artificial intelligence system, as it exists today, is simply a computer that has been programmed to recognize patterns in data and then loaded with huge data sets.
How AI Differs from Traditional Computing
The result of this is that AI systems can process information that isn’t formatted with a strict structure. For most of computing history, humans could only give instructions to computers via programming languages. Now, due to AI, computers can use Natural Language Processing (NLP) to follow your instructions the way you type or speak. Where traditional computing systems needed exact instructions, AI-enabled systems use their “experience” recognizing patterns in writing to interpret the nuance of our language and take the action you’re requesting, even if you can’t communicate it in a strict and technical manner.
A good way to understand the difference is by looking at how traditional programming works. In conventional computing, a developer must write explicit instructions that a computer follows step by step. If a program is designed to categorize emails, for example, it would need a strict set of rules: “If the email contains the words 'Congratulations' and 'You won,' mark it as spam.” This rule-based system is effective but rigid. AI, on the other hand, learns from patterns in data. Instead of being explicitly told how to categorize emails, an AI model can be trained on thousands of emails labeled as spam or legitimate and learn to recognize the common characteristics that distinguish them.
AI's Pattern Recognition and Learning Capabilities
Let’s use an example. Programmatically speaking, is this a cat?
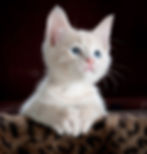
Imagine trying to define a cat in a way that a traditional computer program could recognize. You might attempt to list attributes: a certain size range, fur color, number of legs, ear shape, and so on. But there are countless variations of cats, and describing them with rigid programming rules is nearly impossible. You didn’t learn how to measure whether something is a cat; instead, you saw a wide variety of cats as a baby or toddler until you could tell them from the other animals because you figured out some patterns. AI allows computers to learn in a similar way.
AI models trained on image recognition work by processing thousands, if not millions, of images of cats. Over time, they learn to identify the patterns that make a cat distinct from a dog, a rabbit, or a random background object. The more images the AI sees, the better it becomes at identifying new ones, even if they are from angles or lighting conditions it has never encountered before.
AI in Data Analysis
Now, let’s apply that same learning capability to data. If your business, school project, or any other activity gives you a lot of data, traditional computing requires you to develop a hypothesis to test. With AI, the system you’re using can look for any pattern, not just the ones you think to test for. Imagine a year’s worth of sales data and how you can process it. You might find that recreational products sell more on Friday or Saturday, or that overall sales go up on common paydays in your area.
An AI system, however, might be able to identify subtle variations that allow for nuanced projections. It might detect that a particular product sells well during specific weather conditions or that certain demographic groups exhibit purchasing behaviors that weren’t immediately obvious. If your business needs to make purchasing decisions based on projections, having those projections based on every statistically relevant data point that your system can identify will give you a huge advantage.
AI's Expanding Role Across Industries
While AI is often associated with consumer technology, such as chatbots and virtual assistants, its applications extend across virtually every industry:
Healthcare: AI-powered diagnostics analyze medical scans faster and more accurately than human doctors in some cases. Predictive analytics can also identify at-risk patients based on historical data.
Finance: AI models help detect fraudulent transactions by recognizing unusual spending patterns. They also assist in algorithmic trading, where AI systems make high-speed financial decisions based on market trends.
Retail: AI-driven recommendation engines, like those used by Amazon and Netflix, personalize customer experiences by predicting what products or content users are most likely to engage with.
Manufacturing: Predictive maintenance AI can detect machinery issues before they become critical, reducing downtime and saving costs.
Education: AI-driven tutoring systems personalize learning experiences by adapting to students' strengths and weaknesses.
Ethical and Practical Considerations
Of course, AI isn't perfect, and it comes with challenges. One of the biggest concerns is bias in AI models. Since AI learns from data, if that data contains biases, the AI system can unintentionally perpetuate those biases. For example, AI used in hiring could favor certain demographic groups if its training data reflects historical hiring biases.
Another concern is job displacement. As AI automates repetitive tasks, some jobs will inevitably be affected. However, AI also creates new opportunities by freeing humans from routine work, allowing them to focus on creative and strategic tasks.
The Future of AI
AI is constantly evolving, and as technology advances, its capabilities will continue to expand. One of the most anticipated developments is explainable AI (XAI), which aims to make AI decision-making more transparent and understandable. This would help build trust in AI systems, especially in critical areas like healthcare and criminal justice.
Another major area of development is general artificial intelligence (AGI). Unlike today's AI, which is specialized in specific tasks, AGI would have human-like reasoning and adaptability. While AGI remains a distant goal, ongoing research is bringing us closer to AI systems that can think more broadly and flexibly.
Conclusion
At its core, AI is about pattern recognition and learning from data. While it may seem mysterious, the underlying principles are relatively simple. By enabling computers to process unstructured information, AI has transformed how we interact with technology and how businesses operate. As AI continues to evolve, its potential is only just beginning to be realized.